In recent years, machine learning (ML) has transitioned from a niche area of computer science into a powerful driver of innovation across various industries. This branch of artificial intelligence (AI) involves the development of algorithms that enable computers to learn from and make decisions based on data. The potential of machine learning is immense, and its impact is already being felt in fields ranging from healthcare to finance. In this blog post, we will delve into how machine learning is revolutionising these industries, providing detailed examples and insights into the current and future implications of this technology.
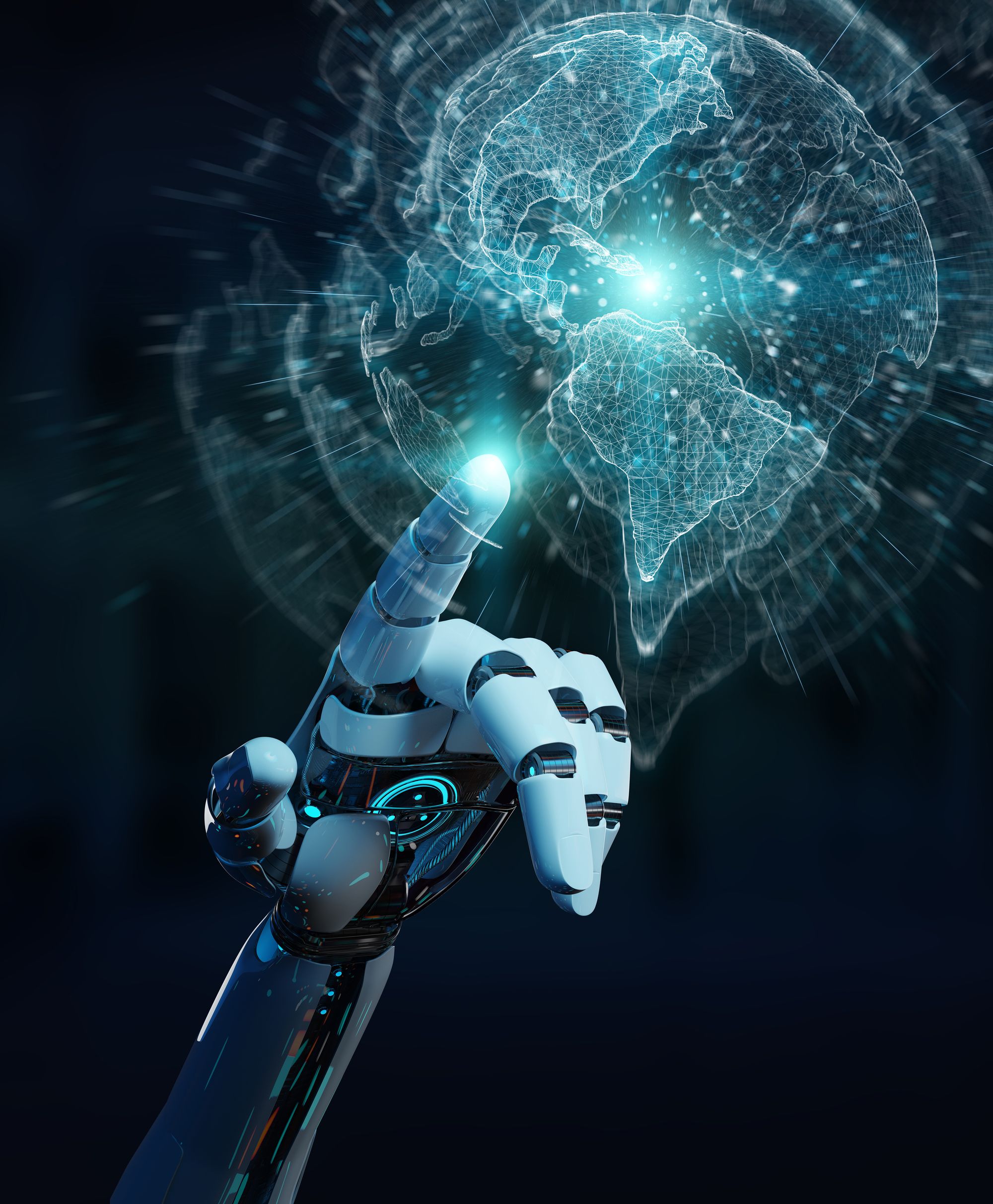
Healthcare
Early Diagnosis and Personalised Treatment
Machine learning enhances diagnostic accuracy and personalises treatment plans by analysing vast datasets, including patient histories and medical images. Google's DeepMind, for instance, has developed an AI system that detects breast cancer from mammograms with greater accuracy than radiologists, enabling early detection and improved survival rates.
Predictive Analytics
ML-powered predictive analytics in healthcare can foresee patient deterioration, hospital readmissions, and the onset of chronic diseases. IBM Watson Health, for example, uses ML to monitor ICU patients' vital signs in real-time, alerting medical staff to potential issues before they escalate.
Drug Discovery and Development
Machine learning accelerates drug discovery by predicting the effectiveness of molecular compounds against diseases. Atomwise utilizes ML to screen millions of compounds rapidly, reducing the time and cost of developing new medications.
Finance
Fraud Detection and Prevention
Traditional fraud detection methods are often rigid and easily circumvented. ML algorithms continuously learn from new data, improving their ability to detect fraudulent activities. PayPal employs ML to analyze transaction data in real-time, identifying suspicious patterns and preventing fraud.
Algorithmic Trading
In finance, algorithmic trading leverages ML to analyze market data and execute trades at optimal times. Firms like Renaissance Technologies use ML-driven strategies that adapt to market changes, consistently outperforming human traders.
Credit Scoring and Risk Assessment
ML enhances credit scoring by evaluating a broader range of data points, such as social media activity and transaction histories. ZestFinance offers alternative credit scoring, enabling better access to credit for individuals with limited histories.
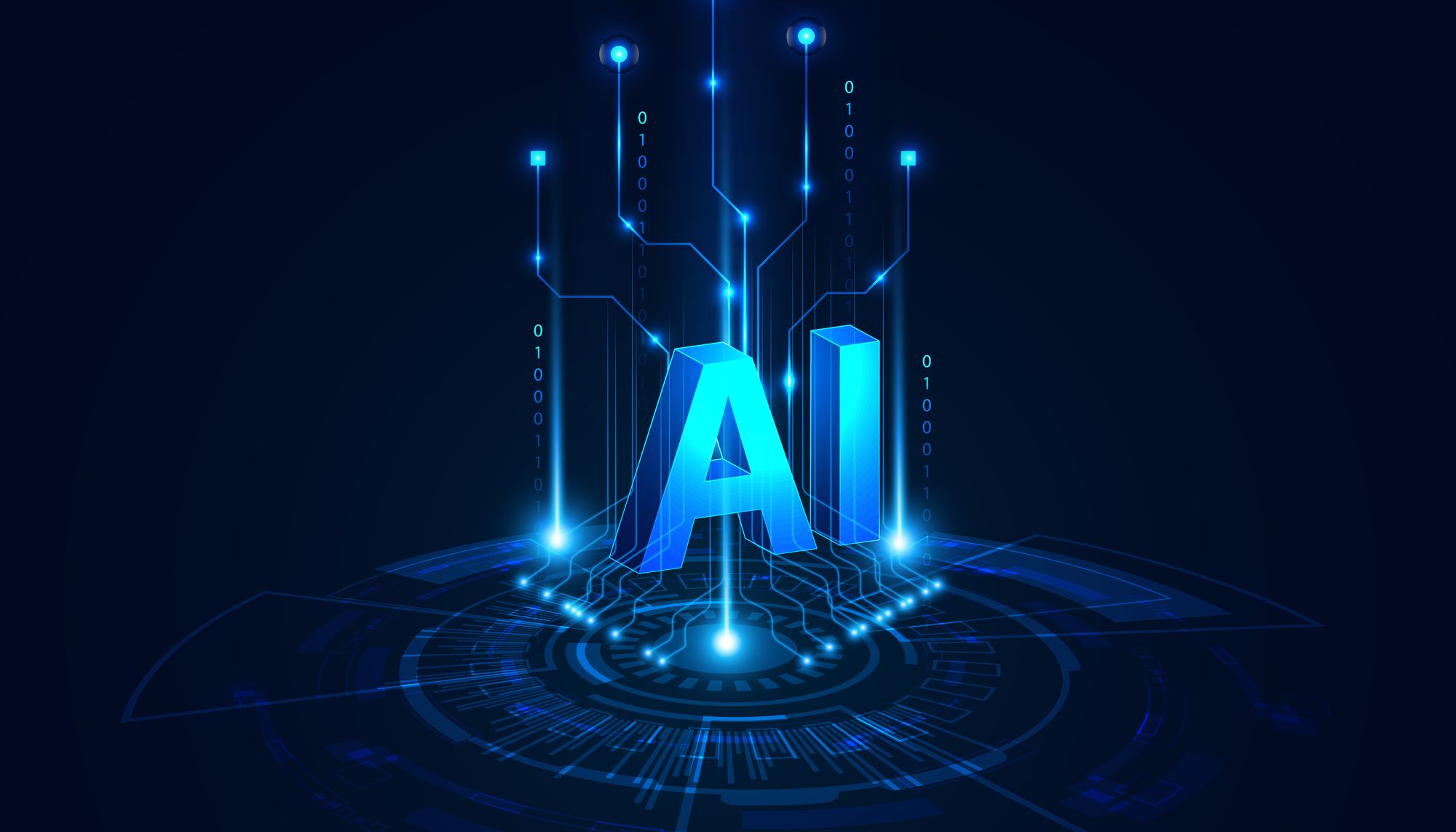
Manufacturing
Predictive Maintenance
Machine learning predicts equipment maintenance needs by analyzing sensor data, reducing downtime and extending machinery lifespan. GE's Predix platform uses ML for real-time monitoring and predictive maintenance, optimizing operational efficiency.
Quality Control
ML improves quality control by analyzing production data and detecting defects with high accuracy. Siemens employs ML in its manufacturing processes, identifying anomalies early to enhance product quality and reduce waste.
Retail
Personalized Recommendations
Retailers like Amazon and Netflix use ML to provide personalized recommendations by analyzing user behavior, purchase history, and demographic data, enhancing customer satisfaction and loyalty.
Inventory Management
ML optimizes inventory management by predicting demand based on sales data and market trends. Walmart leverages ML to ensure products are available when needed, reducing costs and improving customer experience.
Transportation
Autonomous Vehicles
Machine learning is the backbone of autonomous vehicle technology. Companies like Tesla use ML to analyze sensor data in real-time, enabling cars to navigate, make decisions, and improve safety autonomously.
Predictive Maintenance for Fleets
Similar to manufacturing, ML in transportation predicts maintenance needs for vehicle fleets, reducing downtime and maintenance costs. UPS, for example, uses ML to monitor vehicle performance and schedule maintenance efficiently.
Agriculture
Precision Farming
Machine learning revolutionizes agriculture through precision farming, which involves analyzing data from sensors and satellites to optimize planting, irrigation, and harvesting. John Deere's ML-powered equipment helps farmers increase crop yields and reduce resource use.
Crop Health Monitoring
ML algorithms analyze satellite images and sensor data to monitor crop health and detect diseases early. This enables farmers to take proactive measures, ensuring better crop management and higher yields.
Entertainment
Content Recommendations
Streaming services like Spotify and Netflix use ML to analyze user preferences and recommend music, movies, and shows tailored to individual tastes, enhancing user engagement and satisfaction.
Content Creation
Machine learning is also being used to create content, such as AI-generated music and scripts. OpenAI's GPT-3, for example, can generate human-like text, opening new possibilities for content creation in the entertainment industry.
Challenges in Machine Learning Adoption
Despite its transformative potential, the adoption of machine learning is not without challenges:
Data Privacy and Security
With the increasing reliance on data, concerns about privacy and security are paramount. Ensuring that ML systems do not infringe on individual privacy rights or expose sensitive information is critical.
Bias and Fairness
Machine learning models can inadvertently learn and perpetuate biases present in training data. This can lead to unfair or discriminatory outcomes, particularly in sensitive areas like hiring or lending.
Interpretability and Explainability
Many ML models, particularly deep learning models, operate as "black boxes," making their decision-making processes opaque. Explainable AI (XAI) seeks to address this by developing methods to make ML models more transparent and understandable to humans.
Future Trends in Machine Learning
Looking ahead, several trends are poised to shape the future of machine learning:
Explainable AI
As mentioned, the need for transparency in AI decision-making is driving advancements in explainable AI. This field aims to create models that not only perform well but also provide clear, understandable explanations for their predictions and decisions.
Advancements in Natural Language Processing (NLP)
Natural language processing continues to evolve, with models like OpenAI's GPT-3 leading the charge. These advancements are enabling more sophisticated and human-like interactions between machines and humans, opening new possibilities for applications in customer service, content creation, and beyond.
Federated Learning
Federated learning is an emerging approach that allows models to be trained on decentralized data sources without compromising privacy. This technique is particularly promising for industries like healthcare and finance, where data privacy is crucial.
Integration with Internet of Things (IoT)
The convergence of machine learning and IoT is creating intelligent systems that can analyze data from a multitude of connected devices in real-time. This integration is driving innovations in smart cities, home automation, and industrial IoT applications.
Conclusion
Machine learning is transforming industries across the board, driving efficiency, reducing costs, and improving outcomes. From early disease detection in healthcare to personalized shopping experiences in retail, and from autonomous vehicles in transportation to precision farming in agriculture, the impact of ML is profound and far-reaching. As machine learning continues to advance, its potential to innovate and revolutionize industries will only grow, heralding a future where intelligent systems enhance every aspect of our lives. Those who embrace this technology will be at the forefront of a new era of innovation and progress.
Machine learning is not just a technological advancement; it is a catalyst for change that is reshaping the world as we know it. As we move forward, addressing the challenges and embracing the trends will be key to unlocking its full potential and ensuring that its benefits are realized across all sectors of society.